My thoughts on Airbyte
- timandrews1
- Aug 18, 2022
- 4 min read
What a great time to be alive in the data space. There are a plethora of data tools out there to make our jobs easier, at least in most cases. In other cases, the number of tools that we stitch together can have the opposite effect, resulting in a confusing system, which becomes difficult to troubleshoot when something breaks. However, the good outweighs the bad.
Many tools are open-source with a paid-for cloud version, or paid-for version which comes with support. This makes it very easy to experiment and discover something that works well for data teams. Gone are the days when the only way to get your hands on software involved scheduling a demo with the vendor and installing a 14-day trial version (though some vendors still cling to this older practice).
I recently installed Airbyte in my home lab with the aim in syncing some of the Home Assistant data I have stored within my local Postgres database to my BigQuery instance. I do realize that part of the reason for using Home Assistant rather than another automation data hub is to be out of the cloud, but using BigQuery isn't really the same thing as relying on a vendor's proprietary cloud for management of home devices.
I hesitate to describe this post as a review, but rather more of a relaying of my experience in using the software.
Installation
I'm torn on this aspect of Airbyte. Airbyte comes in two installation and deployment methods: cloud or Docker Compose stack. While I do run Docker containers on a regular basis, I wish that there was an option to perform a native installation. Generally speaking, I prefer to spin up LXC containers and install needed software when it comes to a service that I want running 24/7. When running long uptimes, Docker containers seem to require more maintenance and go down more often in my experience.
Regardless, it's very easy to actually perform the install: clone a git repo, cd into that repo, and run the Docker Compose file. The only issue that I had is that I was already using the web UI's port on the host for something else. The installation instructions don't mention anything about this, or how to fix it. It just assumes that you're going to be using port 8000. That could be confusing for those who haven't used Docker in the past.
Establishing database or other end-point connections
Airbyte has a large array of built-in connectors. The setup is very intuitive, and each connector has a custom form built for just that connector. Additionally, a setup guide for your specific connector appears on the right panel of the page while you're setting up your connection on the left. It's a lot easier than setting up an ODBC connection for newer cloud data warehouses. Those dialogs often contain a huge list of fields, some of which may or may not be needed, based on prior fields. Airbyte isn't like that. The BigQuery connector even guided me to the appropriate permissions needed for my service account.
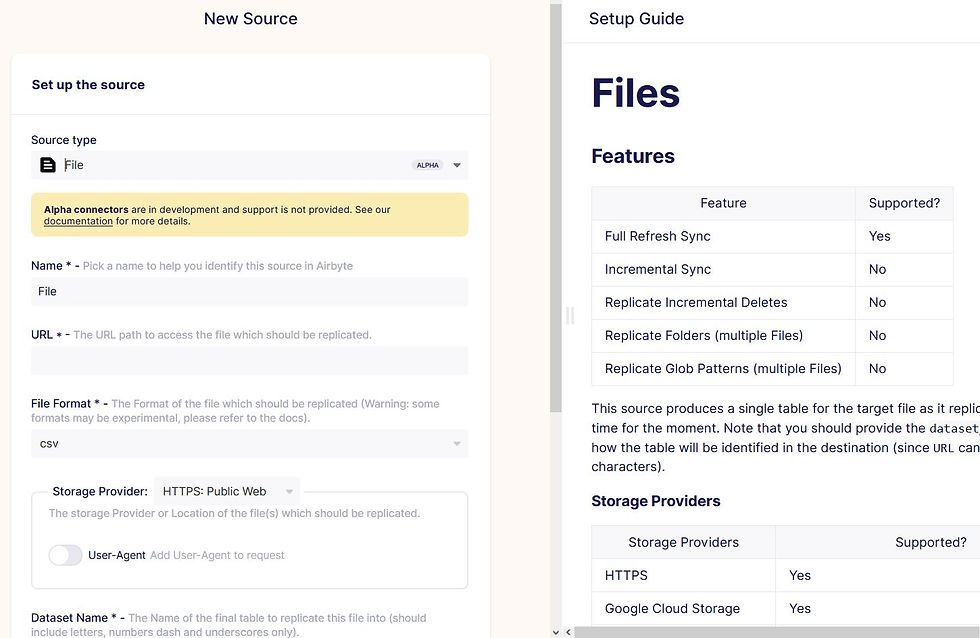
In particular, configuring a BigQuery destination to stage files in Cloud Storage before importing to BigQuery was very streamlined and easy to set up. Most of my work happened on the Google Cloud side -- creating a new service account, storage bucket, dataset, and assigning permissions.
Management and security
Airbyte's UI is very simplistic. There isn't much to do. I'm all for simplicity. One issue that people run into is authorization - there is none. Anyone who can get to the web UI on the network can change things in the Airbyte installation. In a way, that's no different than the old days when web.configs were stored on the web server, and anyone with access to the server could get at those connection strings. However, at least in this case, no one can see passwords. But, in theory, someone could log on, create a new destination to their own database, and then start streaming from a pre-configured source.
Airbyte's first recommendation on is to use firewall rules to restrict the client IPs that can hit the web UI. I think this is a workable solution, especially for a home lab. The other recommendation is to use a reverse proxy with authentication on it, which will then be allowed to communicate with Airbyte. That solution is better, and even workable for home labs, as many of us have Nginx set up for reverse proxies already.
Still, I wish there was a way to integrate authentication out of the box, and allow specific users to manage different sets of connections.
From a debugging perspective - the logs are not intuitive. It's difficult to identify true errors (I ran into some with my authentication roles in Google Cloud - but they were obscured).
Conclusion
I really like Airbyte and would recommend it. It's very simple to set up, when compared to some other tools such as just using vanilla Kafka. I chose to stream two of my larger Postgres tables, just using delta extracts rather than CDC as that was not necessary in my case. It works like a charm. My next task will be to stand up Prefect to chain dbt tranforms to the end of Airbyte streams.
Comentarios